Logistic Regression
15 Nov 2018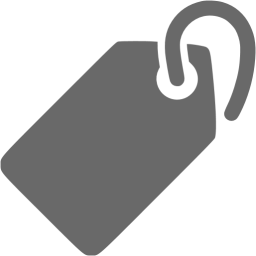
-
Logistic Model is a statistical model that uses a logistic function to classify dependent variable.
-
In general, (Binary) Logistic Regression is used for binary classification. (Pass/Fail, Alive/Dead, Win/Lose, etc…) If there are more numbers of dependent variables, you should look into multinomial logistic regression
-
As its purpose is mainly in binary classification, the model is often used for supervised machine learning.
-
The dependent variables are labeled as 0 or 1.
-
If the probability of predicting dependent variable to 0 is above 50%, the dependent variable will be classified as 0. Else, it would be classified as 1.
The Logistic Model derived from linear regression. If we set y as the probability of predicting one of the two dependent variables, classification becomes easy.
If y > 0.5, it will be labeled as dependent variable A. If y < 0.5, it will be labeled as dependent variable B.
\[y = ax+b\]However, as we can see from the linear regression model above, y and x can have infinite values. As the name suggests, the logistic model is derived from the linear equation by transforming it with logit function.
Logit Function
Logit function, or log-odds is the logarithm of the odds(relative probability the event will happen)
\(odds : \frac{p}{1-p}\) p is the probability that the event will happen. Thus, the logit function and inverse logit function is:
\[logit(p)= ln\frac{p}{1-p}\]Logistic Function
Using the logit function, the linear model can be transformed as following equation:
\(logit(p)= ln\frac{p}{1-p}= ax + b\)
\(\frac{1-p}{p}= \frac{1}{e^{ax+b}}\)
\(p= \frac{e^{ax+b}}{e^{ax+b}+1}\)
How to Use it on Python
Let’s get into some practical stuff now. The code for logistic regression is pretty short and simple. You just need training dataset and testing dataset to build a model.
from sklearn.linear_model import LogisticRegression
# Calling the function and training data
lg = LogisticRegression() #make an instance of the Model
lg.fit(x_train, y_train) #fit your training dataset
# Making predictions and calculating accuracy
predictions = lg.predict(x_test) # Predictions
accr = lg.score(x_test, y_test) # Accuracy
print(predictions)
print(accr)